Patient Privacy at Risk: The Hidden Flaws in Healthcare Data De-identification (And How to Fix Them)
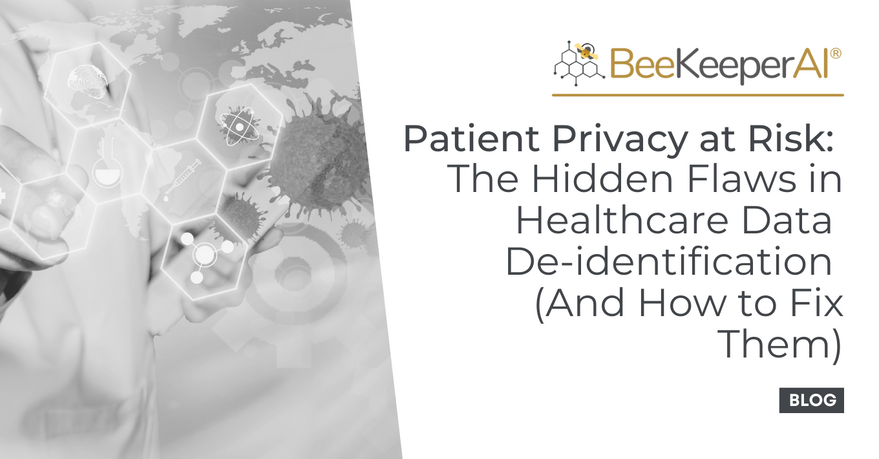
In an era where modifying a single DNA sequence can cure disabling diseases and a retinal scan can reveal important, unappreciated chronic diseases, the three-decade-old practice of data de-identification has become healthcare's equivalent of using a paper lock in a digital world.
While HIPAA revolutionized patient data protection in 1996, today's interconnected digital landscape has rendered these safeguards obsolete.
Every day, healthcare organizations share vast amounts of "de-identified" patient data to fuel AI innovation, operating under the dangerous illusion that removing 18 specific identifiers makes patient data truly anonymous.
But in a world where artificial intelligence can cross-reference thousands of data points in seconds, and where social media footprints create digital shadows of our personal lives, this assumption isn't just outdated—it's putting millions of patients at risk.
The healthcare industry stands at a critical crossroads:
→ Continue relying on inadequate privacy protections that were designed for the dial-up internet age
OR
→ Embrace emerging technologies that can actually deliver on HIPAA's original promise of protecting patient privacy while advancing medical innovation.
HIPAA: The first pass at patient data privacy protection
Almost 30 years ago, in the early days of electronic health records and the internet, and before the smart phone and social media, the Department of Health and Human Services (HHS) issued the Standards for Privacy of Individually Identifiable Health Information ("Privacy Rule") to implement the requirements of the Health Insurance Portability and Accountability Act of 1996 ("HIPAA").
Enforced by the HHS Office of Civil Rights (OCR), the Privacy Rule established a set of standards that control the use and release of “protected data” by “covered entities” intended to protect patient data and privacy.
The rise of de-identified patient data
To avoid negatively impacting biomedical research, the Privacy Rule carved out exceptions for use of protected health information (PHI) in research, as well as the use of limited data sets, and determined that de-identified data was not considered PHI.
These exceptions have become widely utilized as data-driven research has become more prevalent, especially with the advent of artificial intelligence.
Some care delivery systems have developed de-identified versions of their patient data sets to support data-use activities, and others have joined commercial ventures that de-identify the PHI and then offer it to industry for use in a variety of activities, including the development of AI models and AI powered applications.
The changing tech landscape has reduced the privacy protection of data de-identification
The rise of social media and proliferation of individually identifiable data available on the internet and through third-party data aggregators has markedly diminished the privacy protection provided by data de-identification and fundamentally changed the risk to patient privacy.
In fact, in a 2018 paper published in Nature, researchers demonstrated that 99.8% of patients from a de-identified data set could be re-identified with only 15 demographic attributes.
Additionally, since the Privacy Rule holds that de-identified data is not considered PHI, the related privacy protections under HIPAA are lost once a data set is de-identified.
As a result, there is no protection or recourse if de-identified data is re-identified by a third party that is not a HIPAA defined Covered Entity and then used for nefarious purposes such as identity theft or healthcare fraud.
These concerns have led the EU to move beyond HIPAA with data privacy, mandating that de-identified data cannot be re-identified.
Data de-identification excludes important data types for clinical AI
Several important data types cannot be de-identified or may be too risky or time consuming to utilize de-identification to protect individual privacy, including some genomic data, retinal and iris images, imaging/video data, and even social determinants of health.
De-identified data impairs AI performance
In some instances, the de-identification process can render the de-identified data not fit for the intended purpose. For example, if an algorithm was developed to predict outcomes based on time/date of service, but the dates were randomly modified to obfuscate dates of service as part of the de-identification, the algorithm performance can be compromised by the deidentification process.
>>> Several experts have published on the importance of real-world data in evaluating AI models.
New technologies eliminate the need for data de-identification
A new class of “privacy enhancing technologies” and platforms are now available that provide far more robust patient data protection with improved data fidelity and usability, without the substantial expense and time commitment required for data de-identification.
For example, confidential computing platforms can provide complete data protection via end-to-end encryption and secure computing enclaves, eliminating the need to transfer data to third parties.
Confidential computing allows healthcare delivery organizations to
1) keep the data within their HIPAA-compliant, protected data environment with the added protection during the computing cycle,
2) safely leverage their data assets for internal and extramural research projects (including industry funded projects), and
3) protect the intellectual property of the AI developers.
Importantly, with these technologies, AI developers work with unadulterated, real-world patient data, which provides more reliable model performance and meets regulatory requirements for model and application performance validation on real-world data.
What About Federated Learning Platforms or Secure Multiparty Computation?
Federated Learning platforms also eliminate the need to transfer data outside of the data holder’s protected environment but can be attacked and are subject to data leakage in the training weights and parameters.
Additionally, federated training does not in itself protect AI model IP.
Combining federated learning with confidential computing to create secure federated learning is now possible and can resolve these challenges.
Secure Multiparty Computation can also protect the data and algorithms with advanced data and model encryption but requires higher levels of collaboration and coordinated interactions between data holders and algorithm developers which can be challenging in complex, resource constrained healthcare environments.
It’s time to upgrade our privacy approach to PHI in Healthcare AI
Here are some next steps to consider:
To Regulators:
To Industry Leaders, Physicians, Executives, and Patient Advocates:
EscrowAI™ is a secure collaboration platform where an algorithm is protected as it is sent by the algorithm owner to computer on curated, encrypted, information in the data provider's environment. The best part – no one sees either the sensitive data or the algorithm IP.
Mission driven AI development and deployment
Security for sensitive protected data and intellectual property
Increased efficiency and accelerated innovation.
0 comments
Leave a comment
Please log in or register to post a comment