Generative AI Misses the Mark in Healthcare – What It Needs to Succeed
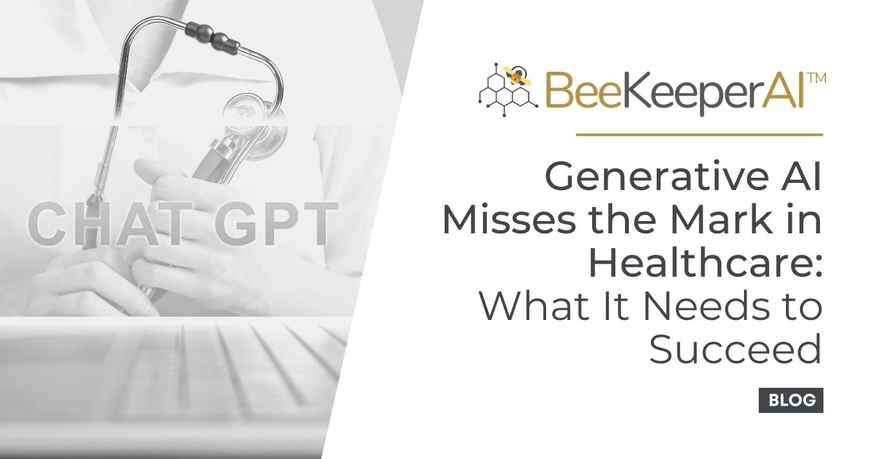
By Michael S. Blum, MD, CEO of BeeKeeperAI™
Key Points:
- ChatGPT is impacting industry and society in unprecedented ways
- The opportunity for GPTs to improve healthcare delivery is enormous, but the technology is immature and not sufficiently reliable for general healthcare use
- The AI models need additional training on real-world healthcare data to perform adequately in healthcare, but accessing that data is challenging due to patient privacy concerns
- New confidential computing technologies are now available to protect patient data and the AI models, creating a pathway for Generative AI success in healthcare
The advent of OpenAI’s ChatGPT3 (GPT3) Artificial Intelligence (AI)-powered chatbot sparked an unprecedented societal appreciation for the power of AI. While AI has been broadly deployed across industries for a decade, it remained mostly hidden from the typical user. The release of GPT3 in late 2022 changed all of that. Suddenly, a user with minimal computer literacy and no programming or data science training whatsoever could ask an AI-based application to create a response to a question in simple everyday language, regardless of complexity of the underlying subject matter. It was mind-blowing and ignited a societal interest in the AI space and its potential impact on commerce and society in general. It was not long before patients, clinicians, and researchers began exploring GPT3’s capabilities in healthcare.
The large language model (LLM) that powers GPT3 was trained on the vast array of information and language across the open internet. As a result, it is ready to respond to an almost infinite variety of questions or prompts. However, as researchers began experimenting with ChatGPT’s performance in complex reasoning or analytical tasks in engineering, science, and medicine, lower levels of performance became apparent (1,2,3), and the phenomenon of AI “hallucination” was observed (4,5).
GPT Needs to Learn How to Say “IDK”
Chatbots and apps powered by the most recent LLMs have demonstrated impressive capabilities in summarizing vast information and creating natural language text in response to queries in the healthcare domain. ChatGPT4 has been reported to have “passed the Boards”, answering exam questions correctly 90% of the time6. However, in real world clinical scenarios, they have also been shown to make important errors, creating significant concerns for their use in clinical practice (5,6). Given that these GPT base models have been trained on the open internet with limited real-world healthcare data and lack the reasoning capabilities of the human brain, it is unsurprising that they struggle with the complexity and nuance of real-world clinical scenarios which contain edge cases described in patient medical records but not often spoken to in published information such as textbooks, clinical trials, or clinical guidelines.
Additionally, GPT’s tendency to “hallucinate” when unable to answer a question based on prior training is particularly problematic in healthcare. Rather than indicating its inability to provide a recommendation with sufficient confidence, it essentially “makes up” an answer and present it in very authoritative sounding text - the worst possible scenario for a clinician relying on a technology for support.
While LLMs will soon play a significant role in addressing highly defined, predictable, and redundant operational and administrative processes in healthcare, they will require significant, additional training on large volumes of high quality, diverse, representative, real-world healthcare data before they can be a trusted clinical partner. These data are held by care delivery organizations, patients, payers, academia, and industry (bio pharmas, contract research organizations, healthcare device vendors, etc.). Early examples of additional training of the LLM base models have shown promising results with improved performance. However, these data are exceedingly difficult to access due to patient privacy concerns and regulatory barriers. Importantly, GPTs will also require additional engineering to learn “humillity” and to learn when and how to say, “I don’t know”.
GPT Doesn’t Understand the Risk of PHI
By the nature of the LLMs, during the training process, they “memorize” some of the data on which they learn7. For publicly available data this memorization is not an issue – however, for extremely sensitive, confidential data, it is a serious legal and ethical challenge. Access to protected patient health information (PHI) during model training, which is then exposed to others without the individual patient’s consent can result in serious harm to patients and severe legal and regulatory consequences to the healthcare delivery organizations. This same concern about exposure and loss of protected health information has hindered the development of clinical AI prior to the arrival of GPT/LLMs and has dramatically retarded the penetration of AI-based technologies into healthcare as compared to other industries. Research reveals that over 90% of healthcare AI remains in research and development due to challenges accessing the real-world data required for regulatory or market validation.
Approaches such as de-identification (anonymization) and creation of synthetic data sets will be useful in early training exercises, but, at some point, access to real-world, protected health data will be required to develop clinically relevant, generalizable, and reliable models. The concerns over inadvertent exposure of PHI contained in an LLM are not theoretical – user input prompts containing PHI are saved and available to the provider of the LLM, and the LLMs themselves have been shown to memorize complex data during the training process (7,8). We must also appreciate the more typical cyber risks as a bug in OpenAI code previously exposed not only GPT queries, but also private user account information including credit card information (9,10). Fortunately, contemporary technologies including secure, confidential computing enclaves and privacy preserving platforms are now available that allow training and deployment of the LLMs in environments that eliminate these data privacy and security risks (11,12).
An Rx for Generative AI to Succeed in Healthcare
Generative AI and LLMs are still in their infancy and rapidly climbing the “hype cycle”. Increasingly large and intensively trained base LLMs will undoubtedly impact healthcare technology and delivery. Apps and applications powered by these technologies hold promise to help clinicians deliver higher quality care more efficiently while simultaneously ameliorating the provider burn-out crisis. They are already employed in ambient conversation recognition applications to automate the note creation process. LLMs will evolve and their output will improve over time as they mature. Access to large volumes of real-world PHI will be critical to developing models that can make reliable and accurate clinical recommendations that generalize across populations without bias. While access to the PHI is challenging and will require protecting the data during training and protecting the LLMs themselves during deployment to prevent privacy breaches, new confidential computing platforms that will meet these needs are evolving in parallel.
Harnessing the immense potential of LLMs in healthcare delivery will require BOTH a robust ecosystem of PHI-accessible data AND confidential computing platforms to protect the data and the LLMs. With our partners, we are leading the way to build this infrastructure, which will dramatically improve the quality, cost, and experience of patient care.
References
- Will ChatGPT transform healthcare? | Nature Medicine
- Levine, D. M. et al. Preprint at medRxiv (2023)
- After failing IIT JEE entrance exam miserably, ChatGPT fails to beat humans at accounting: Know more details (msn.com)
- Hallucinations Could Blunt ChatGPT’s Success - IEEE Spectrum
- Benefits, Limits, and Risks of GPT-4 as an AI Chatbot for Medicine | NEJM
- Can ChatGPT Be a Doctor? Bot Passes Medical Exam, Diagnoses Conditions (insider.com)
- Copilot suggested API Key as well · community · Discussion #21267 · GitHub
- Compromising LLMs using Indirect Prompt Injection (Github)
- ChatGPT and large language models: what's the risk?
- ChatGPT bug temporarily exposes AI chat histories to other users - The Verge
- Securing Healthcare AI with Confidential Computing (beekeeperai.com)
- INTEL OPTIMIZED CASE STUDY SERIES: BeeKeeperAI Secures AI Algorithms with Infrastructure from Intel, Microsoft, and Fortanix
0 comments
Leave a comment
Please log in or register to post a comment